How to make strident predictions about predictive analytics
Image from Shutterstock.
Being able to make predictions is crucial for lawyers. Let’s explore the nature of judicial prediction-making by attorneys and see how the latest in artificial intelligence-powered legal technology comes into play in this altogether ubiquitous, yet highly formidable task.
On a macroscopic scale, clients want to know your erstwhile prediction concerning how their legal case will turn out. In finer detail, you might be stridently attempting to predict whether a judge will grant your recent motion or how the court might rule on a potential objection that you intend to lob while in the heat of a courtroom battle. All of these legally steeped activities entail having to assess the likelihood of a future event or outcome.
How do attorneys make these heady predictions?
The most prevalent approach would seem to consist of lawyering intuition.
Another approach for making legal predictions consists of crowdsourcing. Some law offices make use of email threads to carry out group-oriented legal prediction-making.
Using computers to perform legal predictions can extend far beyond being a mere communications forum. A longstanding and ongoing effort to leverage computing for Legal Judgment Prediction serves as the holy grail for those who seek to computationally derive legal forecasts. The general idea is that an app can be developed that will produce legal outcome predictions.
The latest in AI-powered legal technology attempts to exploit AI techniques and technologies to further advance the aspiration of generating legal predictions.
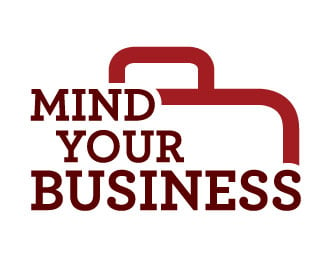
How the AI works
Let’s ensure that we are all on the same page about the nature of contemporary AI.
There isn’t any AI today that is sentient. We don’t have this. We don’t know if sentient AI will be possible.
Today’s AI is principally known as “machine learning,” a form of souped-up computational pattern matching. The standard approach is that you assemble data about a decision-making task. You feed the data into a selected set of machine-learning computer models. After finding mathematical patterns, if so found, the AI system then will use those patterns when encountering new data.
In a legal domain context, the type of data used for crafting machine learning for performing predictions generally consists of two major categories:
• Legal data
• Nonlegal data
The legal data that might be used could consist of past cases and their rendered judgments. Each of the cases would usually be typified by the main characteristics of the given case, such as whether the case was criminal or civil, federal or state, etc.
Nonlegal data is considered to be information that seemingly is outside the realm of legal specificity per se. For example, suppose we collect data about each judge of a case that includes the law school they attended, the number of years on the court, and personal characteristics, such as age, gender and race.
The business of making apps or AI-powered legal technology that can undertake judicial predictions based on such collected data can be a dicey affair.
In June, Gavelytics, a relatively well-known legal analytics firm that had been underway for an inspiring six years, announced it was shutting down. Meanwhile, as their doors closed, Pre/Dicta, a new startup, opened its doors this month and asserts that its product uses the latest in data science for spotting decisional patterns of federal district judges in commercial cases. Besides using docket data, the firm touts that it includes data such as the net worth of the judge, whom they are married to, where they worship and the like. A claimed “86% accuracy rate” is asserted on the company website.
Legal tech is a tough business to be in. The ecosystem of apps for lawyers and law practices is akin to a Darwinian gambit of legal tech that comes and goes—some surviving and others not.
It is, as they say, a cruel world.
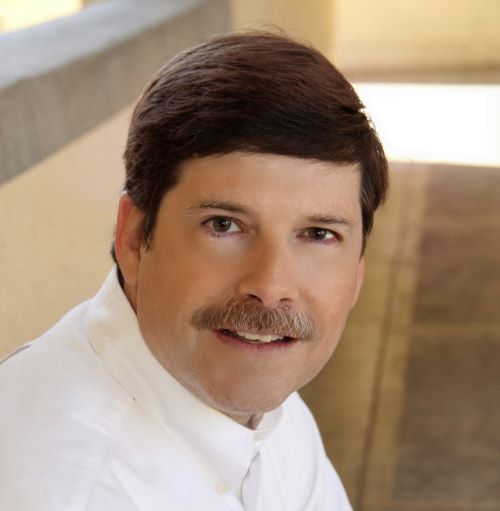
Predicting the future of AI-based legal predictions
With the aforementioned background, let’s do a quick crystal ball look at the future.
Here are some key considerations:
• Claims of prediction rates. Be cautious about decreed high prediction rates. There are ways to perform training and tests when doing AI machine learning that will egg on the prediction outcomes. For example, some researchers got into hot water after claiming a 95% prediction rate on their apps that forecast SCOTUS case outcomes, which is often questionably based on rejiggering historical data and has been criticized as being statistically misleading.
• XAI missing in action. Some AI legal tech that does LJP doesn’t provide a computer-derived explanation for how the prediction was made (this type of feature is known as XAI or explanatory AI). You are entirely left in the dark as to what factors the AI used to render the prediction.
• Don’t forget France. You might vaguely remember that in 2019, a new law was passed in France that banned or made it illegal to use certain forms of judicial analytics, partially undertaken as a result of decried privacy intrusion concerns on behalf of judges. Should these predictive models be using intrusive data on gender, race, age and other personal facets of judges? Though the U.S. would seem unlikely to similarly outlaw such use, the odds are that outsized ethical concerns will be raised if this starts to become prominently featured in the American marketplace.
• Making it real-time. Most of the AI legal tech for LJP is based on historical data. A cutting-edge approach infuses real-time data into predictive efforts. You might liken this to the famous trope that a judge purportedly makes their judicial decisions based on what they had for breakfast that morning (smarmily known as “gastronomical jurisprudence”). If that’s how things really work, the AI has to be up-to-date moment-to-moment.
• Add a strong dose of AI legal reasoning. Almost none of the existing LJP includes the devout legal reasoning that underlies the cases. The cases are only inscrutable blobs that are represented via a series of high-level factors. Efforts are underway to use AI-based natural language processing and advances in large language models to do the nitty-gritty legal analysis of the text of legal cases. By using AI legal reasoning, LJP would be significantly improved as a result of taking into account the particulars of legal cases such as the underlying legal arguments and legal precedents that the cases depend upon. This is a key initiative of my AI legal tech lab and our aim is to augment legal tech with AI legal reasoning innovations for producing heartier legal predictions.
Attempts to use the latest in AI and legal tech to try and make legal-oriented predictions are assuredly going to continue and speedily gain momentum as a means of garnering a competitive edge over your legal competition. Some believe that any savvy lawyer not using such LJP tools could eventually be vulnerable to legal malpractice. Either way, the future is bright for AI legal tech, which can dutifully and accurately aid in legal judgment predictions.
You can take that prediction all the way to the bank.
Lance Eliot is a Stanford fellow at the Stanford University Center for Legal Informatics, a joint affiliation of the Stanford Law School and the Stanford Computer Science Department, and he is the founder and CEO of Techbrium Inc.
Mind Your Business is a series of columns written by lawyers, legal professionals and others within the legal industry. The purpose of these columns is to offer practical guidance for attorneys on how to run their practices, provide information about the latest trends in legal technology and how it can help lawyers work more efficiently and strategies for building a thriving business.
Interested in contributing a column? Send a query to [email protected].
This column reflects the opinions of the author and not necessarily the views of the ABA Journal—or the American Bar Association.